Data Lifecycle Management: Definition and Stages
Every unit of data goes through a lifecycle. Data lifecycle management (DLM) provides structure to the life of data, from acquisition to use and eventually to decommissioning. As the volume and velocity of data growth continue, data lifecycle management becomes imperative.
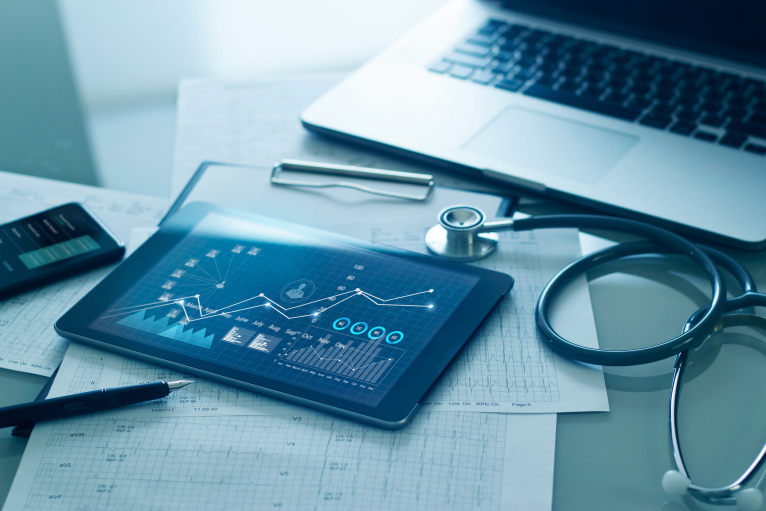
The ability to extract value from data is predicated upon access to it. To be of value, data has to be of high quality and highly accessible. While value is a priority, keeping data from causing harm is critical, and is also addressed by data lifecycle management.
Once created, data must be protected from unauthorized use. It must also be destroyed when it is no longer needed to reduce the threat surface. From creation to destruction, data lifecycle management best practices offer direction on how to most effectively, efficiently, and securely handle data.
Six Stages of Data Lifecycle Management
Data lifecycle management is a framework that defines the stages that data goes through and provides direction on how to optimize each of those. Policies drive the structure through which data flows to allow for automation of processes.
The main stages in the data lifecycle management process are as follows:
Data Generation
Data is generated, acquired from third parties, and gathered from Internet of Things (IoT) devices or machine learning systems. During the data generation stage, it is important to have established rules for gathering data in a standardized format to make it more manageable and accessible.
These rules or policies should take into consideration the different types of data that are collected. Data security and data privacy should also be in place at this stage of data lifecycle management, using tags to identify the category of data (e.g., sensitive information).
Data Storage
The way data is used determines how it should be stored. Content that is in use or needs to be quickly accessible must be protected from unauthorized access and backed up regularly. Data that is not in active use should be archived or deleted. Policies must be in place to define the criteria for where data is stored and when it is deleted.
Data Maintenance
The data maintenance stage of data lifecycle management is where data is organized and curated then continually cared for to keep it accessible and optimized for users. This includes ongoing data correction and verification. Data maintenance also includes ongoing data enrichment and integration.
Data Usage
Once data has been properly processed, it arrives at a critical stage—usage. Data is used across organizations in many ways. It is critical at this stage that data is readily accessible and safe. Rules and processes must be in place to protect data from misuse and mishandling.
Data Sharing
Policies should clearly state what data can be shared and how. This includes how users exchange and access data within an organization as well as how it can be shared externally.
Data Cleaning
This stage of data lifecycle management encompasses policies that direct the deletion and archiving of data when it is no longer actively being used. Data cleaning can save money by reducing the amount of data that is stored, but it is also important for security.
The more data that is stored, the higher the potential risk. Archiving is used when data is no longer active, but needs to be retained for potential future use or to comply with regulations.
Ongoing Requirements for Data Lifecycle Management
Throughout the stages of data lifecycle management, there are three must-haves in terms of day-to-day operations related to data:
- 1. Security
- 2. Availability
- 3. Structural integrity
Each of these should be top of mind when moving through the stages of data lifecycle management. The ultimate goal of the data lifecycle stages is to extract the maximum value from data by creating and maintaining quality data and implementing processes to make it easily accessible while protecting it.
A Brief History of Data Lifecycle Management
Technologies for storing data have been around for longer than many would guess. Nearly in parallel with the adoption of these technologies was growth in data volume and as a result, the management of that data throughout its lifecycle.
Here is a quick review of technology for storing data, ultimately the drivers behind data lifecycle management:
- Mechanical punch card
Invented in 1890, it was first used to tally the 1890 census. - Magnetic tape
Invented in 1928, magnetic tape was originally used to record audio. Its use was expanded to storing data in 1951 with the invention of the UNISERVO I, which was implemented in the UNIVAC I computer. The UNISERVO tape drives provided read and write functionality at a rate of 12.8 KBps and could store up to 184 KB of data. - Hard disk drive (HDD)
First shipped by IBM in 1956, the hard disk drive was designed to work with the IBM 305 RAMAC mainframe computer. It allowed businesses to record and maintain data in real-time and provided the ability to randomly access any record. - Virtualization
CP-68 was the first virtual machine operating system. It was designed for IBM’s System/360. - Solid-state drive (SSD)
The first solid-state drive was released in 1976 by Dataram. It was able to hold up to eight memory boards with 256KB of RAM chips. - Software-defined storage (SDS)
The concept of software-defined storage was born in the 1990s. Software-defined storage provided data storage software for policy-based provisioning and management of data storage that was hardware independent. - Cloud storage
Created in the 1960s, cloud storage was more commonly used by the late 1990s. By the late 2000s, cloud storage service providers, cloud computing, and SaaS became mainstream.
Data lifecycle management has its origins in the 1980s with the introduction of random-access memory storage (RAM) and the transition from mechanical punch cards and magnetic tape storage to databases. As data storage costs dropped, data volumes grew.
Hence the trouble began. Issues quickly arose, such as data duplication, poor data quality, security challenges, data backup, recovery difficulties, and the overall chaos of too much data with too little file organization.
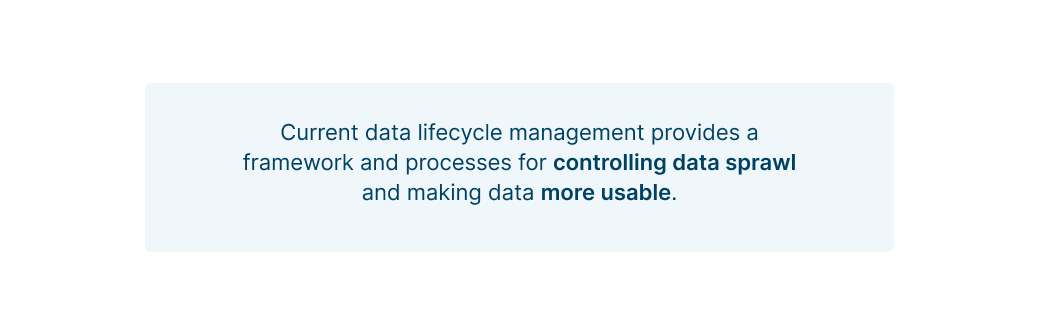
Data lifecycle management continues to evolve to meet the requirements of big data.
DLM vs. ILM
Data lifecycle management (DLM) is often used synonymously with information lifecycle management (ILM), but there are differences; the main difference is:
- DLM focuses on the data as a mass throughout its useful life, helping to make the most recent and useful data accessible quickly and efficiently.
- ILM focuses on what is in the records to help keep all of the data accurate and up-to-date for the record’s lifecycle.
Data Lifecycle Management Benefits
Data lifecycle management provides a framework for clarifying areas of ambiguity related to data, such as:
- Short and long-term storage
- Data backup processes
- Data accessibility when required for legal, regulatory, or other business needs
- Disaster recovery and contingency plans
- Data protection—on-prem and off-prem
- Timing and types of archiving (e.g., accessible archive, cold storage)
- Data retention and disposal policies
Other benefits provided with data lifecycle management include:
- Adherence to compliance requirements for data retention
- Improved access to the right data when it is needed
- Enhanced security
- Increased resiliency in the event of a disaster
- Ability to identify and extract more value from data
- Increased data value
- Controls for data usage
Define Data Lifecycle Management Stages for Better Outcomes
Regardless of size, every organization benefits from data lifecycle management. Data creation and collection are inescapable and ever-growing.
The sooner organizations define and implement the stages of data lifecycle management, the faster the time to savings—time and money. Properly implemented and executed, data lifecycle management processes become integrated into workflows, and the value of data increases.
Egnyte has experts ready to answer your questions. For more than a decade, Egnyte has helped more than 16,000 customers with millions of customers worldwide.
Last Updated: 4th August, 2021
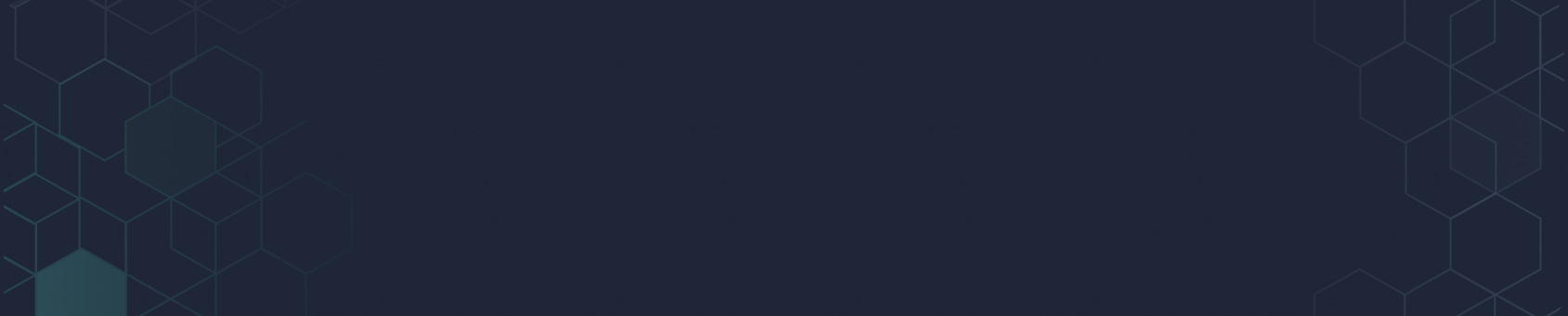